Story - Stritch - AI and Cell Migration

Sasha Demeulenaere, an MD/PhD student in Loyola professor Patrick Oakes’s lab, reviews cell imagery using high-resolution quantitative microscopy. (Photo: Lukas Keapproth)
The art and science of cells: Loyola's innovative approach to understanding cellular behavior and health
The Oakes Lab tackles the physics of life in its study of cell migration
Until Loyola professor of cell and molecular physiology Patrick Oakes collaborated with physics colleagues from the University of Chicago a few years ago, there was no known systematic strategy that could infer the large-scale physical properties of a cell from its molecular components.
Such a strategy is essential to better understanding various cell processes like adhesion and migration, which could improve treatments for cancer metastasis, wound healing, and immune response.
Through their innovative interdisciplinary approach combining biology, physics, and AI, the team developed a suite of data-driven approaches for biophysical modeling of cellular forces that can shed light on cancer, immune response, and tissue regeneration.

Professor of cell and molecular physiology, Patrick Oakes. (Photo: Lukas Keapproth)
The path of discovery
“We are training scientists to be fluent in the languages of both biology and physics,” Oakes says of the graduate and postdoctoral students that conduct experiments alongside faculty.
That fluency is evident in his lab, a hub that combines physics, biology, engineering, and computer science expertise. Surrounded by screens filled with striking, colorful images of cells that can sometimes look more like art than science, it's where faculty, graduate students, and postdoctoral scholars ask—and answer—fundamental questions about how the human body functions at its most elemental level.
Oakes’s team investigates whether cell movement can improve human health. Cell migration is central to processes like mounting effective immune responses and repairing injured tissues. Migration can also contribute to vascular disease, chronic inflammatory diseases, and tumor formation and metastasis. Oakes’ innovative work bridges disciplines and fosters a collaborative environment, exemplifying Loyola's intellectual leadership and commitment to solving complex problems in science and health.
Oakes uses high-resolution microscopy and machine learning to accelerate and expand his research on the cytoskeleton, or the “bones” of a cell. In his lab, researchers combine traditional biological approaches with a number of quantitative approaches, including micropatterning, computational modeling, and optogenetics. His team’s discoveries—understanding cells and developing innovative ways to maximize machine learning—contribute to understanding cell migration and the physics of living systems to develop new approaches to minimize disease.
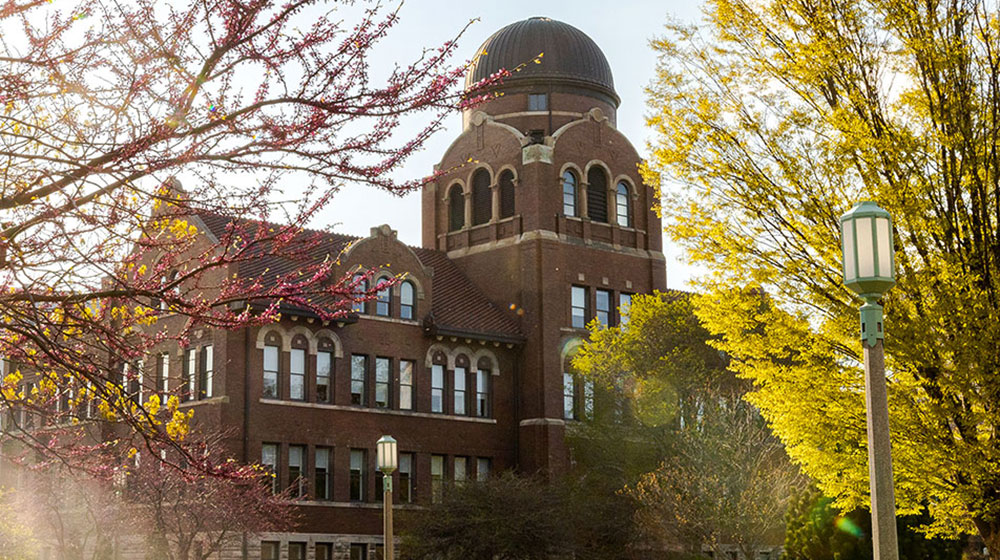
Loyola University Chicago
The Pursuit of Purpose
What can be learned from Loyola’s 153-year track record on social impact
Read the story
College of Arts and Sciences
Bridging ethics and technology: Loyola's pioneering approach to humanizing AI and VR education
An interdisciplinary method of teaching the ethics of AI and VR.
Read the Story
School of Environmental Sustainability
Loyola leads the way in fighting hunger and food waste in Chicago
Initiatives help the environment and the local community.
Read the storyCross-collaboration leads to new insights
“Imagine being given the most complex puzzle you could imagine, with billions of pieces, and no instructions on how to put them together,” says Oakes of the challenge of creating a systematic strategy to infer the large-scale physical properties of a cell from its molecular components.
To tackle this puzzle, Oakes and colleagues from the University of Chicago Department of Physics, along with the University of Chicago's Institute for Biophysical Dynamics and James Franck Institute looked at whether machine learning, a form of artificial intelligence (AI) adept at making connections in overwhelming seas of data, could make predictions to help understand complex processes such as how a cell knows where to adhere and where to pull.
“Microscopy has developed extensively over the previous decades, expanding in both resolution and speed, to produce better images,” says Oakes. “The challenge has been to extract quantitative data from all of these images. The field has really benefited from the approaches brought from other disciplines, such as physics and engineering, and applied to biological systems.”
The team hypothesized that it could train neural networks to predict the forces generated by cells directly from images of a single protein they labeled with a fluorescent marker. Initial results showed the team that distilling a cell's complex processes down to a simpler model was possible.
Using technology to go deep
Neural networks are often called black boxes because their underlying connections are opaque and too complex for humans to understand. To overcome this problem, the team set out to determine if a neural network could be interrogated to extract information from it.
If they could deduce how machine learning calculated and drew its conclusions, it could extrapolate and apply it to other problems. The team proposed and showed three different ways to extricate information from machine learning's black box.
They confirmed they could feed it the artificial images where the team had altered individual components, one at a time, to identify the features that the neural network found important. They established they could use machine learning to improve existing models by allowing the network to determine the values of the model parameters. Finally, they demonstrated that using an agnostic approach to build a new model from scratch was possible. Together, these findings lay the groundwork for other scientists and labs working with machine learning to understand how AI works in this context – just one way research from Loyola blazes a trail for the next generation of innovators.
“Bringing together skill sets and knowledge from different disciplines is fundamental to advancing our field and it’s how my lab approaches problem solving,” says Oakes. “Science is more fun when done together and we all learn something new.” By pulling together different types of expertise and skill while engaging with cutting-edge technology and experiential learning, the university prepares the next generation of leaders to drive impactful change locally and globally.
Cross-collaboration leads to new insights
“Imagine being given the most complex puzzle you could imagine, with billions of pieces, and no instructions on how to put them together,” says Oakes of the challenge of creating a systematic strategy to infer the large-scale physical properties of a cell from its molecular components.
To tackle this puzzle, Oakes and colleagues from the University of Chicago Department of Physics, along with the University of Chicago's Institute for Biophysical Dynamics and James Franck Institute looked at whether machine learning, a form of artificial intelligence (AI) adept at making connections in overwhelming seas of data, could make predictions to help understand complex processes such as how a cell knows where to adhere and where to pull.
“Microscopy has developed extensively over the previous decades, expanding in both resolution and speed, to produce better images,” says Oakes. “The challenge has been to extract quantitative data from all of these images. The field has really benefited from the approaches brought from other disciplines, such as physics and engineering, and applied to biological systems.”
The team hypothesized that it could train neural networks to predict the forces generated by cells directly from images of a single protein they labeled with a fluorescent marker. Initial results showed the team that distilling a cell's complex processes down to a simpler model was possible.
Using technology to go deep
Neural networks are often called black boxes because their underlying connections are opaque and too complex for humans to understand. To overcome this problem, the team set out to determine if a neural network could be interrogated to extract information from it.
If they could deduce how machine learning calculated and drew its conclusions, it could extrapolate and apply it to other problems. The team proposed and showed three different ways to extricate information from machine learning's black box.
They confirmed they could feed it the artificial images where the team had altered individual components, one at a time, to identify the features that the neural network found important. They established they could use machine learning to improve existing models by allowing the network to determine the values of the model parameters. Finally, they demonstrated that using an agnostic approach to build a new model from scratch was possible. Together, these findings lay the groundwork for other scientists and labs working with machine learning to understand how AI works in this context – just one way research from Loyola blazes a trail for the next generation of innovators.
“Bringing together skill sets and knowledge from different disciplines is fundamental to advancing our field and it’s how my lab approaches problem solving,” says Oakes. “Science is more fun when done together and we all learn something new.” By pulling together different types of expertise and skill while engaging with cutting-edge technology and experiential learning, the university prepares the next generation of leaders to drive impactful change locally and globally.